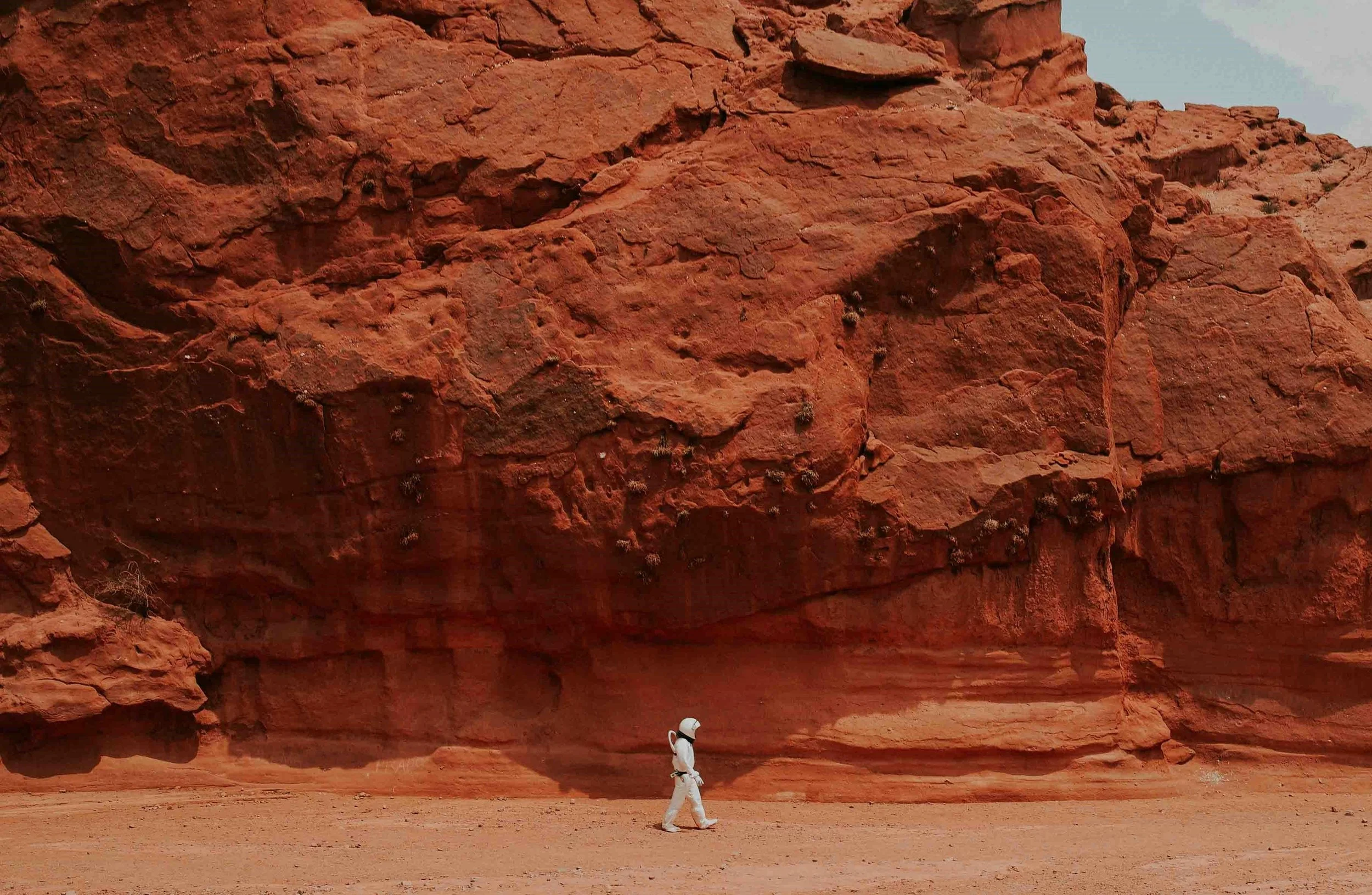
The Science of Rapid Consensus—How Mindhive and AI Are Transforming Collaborative Decision-Making.
By Bruce Muirhead, 23 January 2025
In our interconnected world, collaboration has become the cornerstone of innovation and progress. Whether it’s a small startup iterating on a new product feature or a multinational enterprise devising a corporate strategy, teams must work together swiftly to remain competitive. However, the traditional methods of collaborative problem-solving—extended email threads, lengthy in-person meetings, and multi-layered approval processes—can often stifle creativity and slow decision-making to a crawl.
Recent advances in artificial intelligence (AI) now offer a more efficient alternative. A 2021 survey by the MIT Sloan Management Review found that organizations integrating AI tools into their decision-making processes reported a 30% improvement in both speed and accuracy. Meanwhile, a Harvard Business Review analysis highlighted that teams using AI-based collaboration platforms significantly reduced the time spent in consensus-building, shifting focus toward innovative thinking and action.
This blog delves into how Mindhive’s AI-driven collaboration methodology—referred to as “The Mindhive Way”—utilizes a research-backed, evidence-based approach to accelerate consensus while maintaining a high degree of engagement, transparency, and trust. By combining machine intelligence with human expertise, Mindhive addresses the core challenges of modern collaboration: unstructured communication, disengaged stakeholders, and the difficulty of pinpointing evidence-based insights.
Over the next several sections, we will explore the scientific foundations of collaborative problem-solving, discuss the role of AI in facilitating meaningful engagement, and dissect how Mindhive’s approach ensures swift, informed consensus without sacrificing rigor or quality.
1. The Evolution of Collaborative Decision-Making
Historically, groups have used face-to-face meetings, whiteboards, and roundtable discussions to share ideas and reach decisions. While these methods served as the foundation for teamwork, they often fell short in scalability, especially once projects crossed departmental or geographical boundaries. Virtual collaboration introduced email, instant messaging, and basic project management tools, but these solutions frequently led to information overload, lack of clear accountability, and a phenomenon known as “analysis paralysis,” where teams become stuck in perpetual debate.
Research published in the Journal of Applied Psychology (2019) noted that groupthink—a situation where the desire for harmony or conformity results in suboptimal decisions—became more prevalent when discussions were poorly structured. This aligns with observations that teams without a clear framework or facilitative structure can devolve into echo chambers, stifling dissent and creativity.
As organizations face increasingly complex, multifaceted challenges, from global supply chain disruptions to swiftly evolving consumer demands, speed and quality of decision-making become paramount. A 2020 survey from McKinsey & Company found that companies making decisions quickly were more than twice as likely to report higher profitability and innovation rates. Yet, “fast” decisions do not always equate to “good” decisions unless the team’s approach is guided by data, diverse perspectives, and clear alignment on objectives.
Herein lies the value of AI. Machine learning algorithms excel at sifting through large amounts of data, identifying patterns, and highlighting anomalies far more efficiently than any single human or group of humans. However, AI alone cannot replicate human intuition, contextual understanding, and ethical considerations—critical aspects of any robust decision-making process. Hybrid systems, where AI augments but does not replace human judgment, offer the most promising route. These systems allow human teams to remain at the helm while leveraging data-driven prompts to focus discussions, highlight key insights, and minimize repetitive tasks like note-taking and summarization.
2. Scientific Underpinnings of AI-Human Collaboration
The concept of blending human and artificial intelligence has been extensively studied in cognitive psychology, organizational behavior, and computer science. One of the foundational theories relevant to this blend is the Extended Mind Theory, initially discussed by philosophers Andy Clark and David Chalmers in the late 1990s. They argued that tools and technologies become extensions of our cognitive processes, enabling us to think more broadly, store more information, and tackle problems that would otherwise exceed our mental capacity.
Modern AI-based platforms, such as Mindhive, can be viewed through this lens. When an AI agent collects, interprets, and organizes data for human teams, it effectively extends their cognitive reach. This is especially critical for large-scale projects, where the volume of data—whether it’s consumer feedback, internal metrics, or external market conditions—can be overwhelming. Natural language processing (NLP), a subfield of AI that allows systems to interpret human language, is a key facilitator. By analyzing user comments and discussions, NLP algorithms can:
Identify Patterns and Sentiments: Detect recurring themes or emotional tone in conversations, helping facilitators to gauge consensus or disagreement.
Surface Relevant Content: Automatically recommend articles, reports, or organizational documents that align with the current topic of discussion.
Filter Noise: Highlight the most valuable contributions while minimizing repetition and off-topic tangents.
Additionally, findings from a Stanford University study on mixed-method AI systems suggest that when teams are supported by “explanatory AI”—algorithms that provide rationales for their outputs—trust and effective adoption rates surge. This is crucial because trust is a cornerstone in adopting any new technology, especially one that directly impacts decision-making.
Moreover, the science of collective intelligence underscores the importance of diverse inputs. Studies from the MIT Center for Collective Intelligence have shown that teams with higher levels of cognitive diversity—including varied skill sets, backgrounds, and perspectives—consistently outperform more homogeneous groups on complex tasks. AI can help surface or “spotlight” participants with unique expertise or contrarian viewpoints through algorithms like Mindhive’s Wildcard Algorithm. This ensures a rich tapestry of insights is pulled into the discussion rather than leaving decision-making to the loudest voice in the room.
3. The Mindhive Way: A Research-Backed Framework
The Mindhive Way is not just a tagline; it’s an end-to-end methodology built on:
Structured Ideation & Discussion: Mindhive provides prebuilt templates for brainstorming, strategy sessions, and problem-solving. These templates draw upon best practices from design thinking, collective intelligence, and agile methodologies, ensuring every discussion starts with a well-defined purpose and framework.
AI-Augmented Research: At the core of Mindhive’s approach are Knowledge Discovery Agents (KDA). Once a problem is defined, these agents conduct targeted searches across internal databases and reputable external sources. They then present referenced insights to participants in a chat-like interface, fostering transparency and credibility. Research in the field of information retrieval supports this tactic, demonstrating that citations in AI outputs significantly boost user trust (Chen et al., ACM Transactions on Information Systems, 2020).
Real-Time Highlighting & Insight Generation: Instead of sifting through cluttered chat logs or endless email replies, Mindhive’s algorithms automatically highlight key statements, emergent themes, and potential solutions. This aligns with the principle of cognitive load theory, which suggests that breaking down information into digestible segments enhances working memory capacity (Sweller, Cognitive Science, 1988).
Consensus-Building Tools: Beyond identifying actionable ideas, The Mindhive Way employs voting, ranking, and weighting mechanisms that reflect both AI suggestions and human judgment. This multifaceted approach ensures that the final priorities are both data-driven and aligned with organizational goals.
Automated Consultant-Style Reporting: One of the most time-consuming aspects of collaboration is synthesizing discussions into a coherent document. Mindhive automates this through Large Language Model (LLM)-driven summarization. A 2021 paper in Nature Machine Intelligence explains that well-designed LLM summarization can capture the essence of complex human discussions with over 80% accuracy compared to professional human analysts.
Ethical & Transparent AI: Every piece of AI output includes references to the original data sources, reducing the likelihood of misinformation or so-called AI “hallucinations.” Mindhive also adheres to ISO 27001, GDPR, and SOC2 compliance standards, ensuring data security and privacy—an essential factor in enterprise adoption.
4. Why Rapid Consensus Matters for Organizations
The business landscape is increasingly defined by high volatility and complexity, where delays in decision-making can mean missed opportunities, lost revenue, or strategic misalignment. A study by Bain & Company found that companies making decisions quickly are 40% more likely to remain ahead of market shifts. Conversely, organizations hamstrung by slow, cumbersome processes often see project timelines slip and team morale decline.
Rapid consensus is particularly important in scenarios like:
Crisis Management: In urgent situations (e.g., supply chain breakdowns, product recalls, cybersecurity breaches), swift decisions can mitigate damage and preserve stakeholder trust.
Innovation Cycles: When iterating on new product features or market expansions, a clear and rapid decision-making cycle ensures momentum and competitive advantage.
Cross-Functional Projects: Teams drawn from diverse functions—marketing, finance, operations, R&D—often have conflicting priorities. A structured, transparent approach to consensus ensures alignment and prevents “turf wars.”
However, “rapid” does not always mean “reckless.” Academic research highlights the concept of “bounded rationality,” introduced by Nobel Laureate Herbert A. Simon, which argues that while humans seek to make optimal decisions, our cognitive limitations and information overload force us to settle for “good enough” choices under time constraints. AI-driven platforms like Mindhive help mitigate these cognitive limits by handling data processing tasks, thereby enabling humans to focus on critical thinking and ethical considerations.
Furthermore, employee engagement significantly improves when teams see their inputs lead to tangible outcomes quickly. According to a Gallup poll, engaged teams show a 21% increase in profitability. By rapidly converging on decisions, Mindhive’s approach creates a positive feedback loop: people feel heard, they see their ideas shaping the roadmap, and the organization benefits from a more motivated, aligned workforce.
In essence, The Mindhive Way ensures that speed does not come at the expense of quality, transparency, or inclusivity. Instead, it channels the collective intelligence of diverse stakeholders, guided by AI’s data-driven prompts, to reach well-considered, immediate outcomes.
5. Detailed Steps of The Mindhive Way
To illustrate how a typical Mindhive session unfolds, let’s break down the process step by step, weaving in the scientific rationale behind each stage.
Step 1: Challenge Definition
A clear and concise problem statement sets the stage. Drawing upon research in goal-setting theory (Locke & Latham, Organizational Behavior and Human Decision Processes, 1990), specific and challenging goals tend to lead to higher performance. Mindhive’s platform prompts the facilitator or host to narrow down the key question to ensure every contributor orbits the same objective.
Step 2: Automated Discussion & Survey Setup
Once the problem is defined, Mindhive’s Automated Discussion and Survey Setup Tool recommends whether to proceed with a collective intelligence discussion or a targeted survey. This decision is guided by the platform’s algorithms, which weigh factors like team size, complexity of the issue, and whether quantitative or qualitative input is required. According to a 2020 study in the Journal of Organizational Computing and Electronic Commerce, such automated scaffolding significantly reduces “organizational overhead,” freeing participants to focus on higher-order thinking.
Step 3: AI-Facilitated Exploration
Next, the Knowledge Discovery Agents (KDA) begin a turn-based conversation with the user, refining queries and returning data with citations. This ensures that, from the outset, the discussion is grounded in verified information. The KDA system draws on natural language processing, knowledge graphs, and vector databases to fetch relevant content. The importance of validated, cited data cannot be overstated—numerous meta-analyses indicate that trust in AI is largely shaped by the system’s transparency in how it arrives at conclusions (Schaake et al., Human-Computer Interaction Journal, 2021).
Step 4: Real-Time Collaboration & Engagement
With relevant data in hand, participants enter the discussion phase. Gamification tools—such as badges for active contributors or “insightful” commentary—work in tandem with role-based permissions to maintain a balanced dynamic. Neuroleadership research suggests that recognition and status are powerful motivators, activating the brain’s reward circuitry (Rock, Strategy+Business, 2008). By rewarding meaningful participation, Mindhive creates an environment of psychological safety, encouraging even typically introverted members to share ideas.
Step 5: Insight Highlighting & Ideation
During the conversation, the Insight Generation Algorithm automatically pinpoints statements that diverge from the norm, present novel viewpoints, or build upon previous insights in a unique way. This feature taps into the notion that creativity often emerges at the intersection of different ideas (Sawyer, Group Genius, 2007). By surfacing these intersections in real time, participants can quickly bounce off each other’s suggestions. Mindhive also offers a drag-and-drop Ideation Editor, allowing team members to cluster related insights and form broader solution pathways.
Step 6: Voting & Ranking for Rapid Consensus
Once a sufficient number of ideas have been collected, the system transitions participants into a voting or ranking module. The platform might prompt them to rate each proposal based on feasibility, impact, or alignment with strategic goals. This approach leverages decision science, specifically the Analytic Hierarchy Process (AHP) or multi-criteria decision-making frameworks, to ensure a methodical evaluation (Saaty, Decision Making for Leaders, 1990). The synergy of AI’s data-driven suggestions and human priorities results in a refined shortlist of 6–8 top ideas.
Step 7: Automated Consultant-Style Reporting
After consensus is reached, Mindhive’s Automated Management Consultant-Style Reporting Tool integrates discussion points, ranked ideas, and engagement metrics into a visually appealing report. Each factual statement is accompanied by references, and the overall narrative is generated using advanced LLM-based summarization. This feature responds to a need identified in the International Journal of Project Management (2021), which indicates that lack of clear, actionable documentation is a major risk factor for project delays and misalignment.
Step 8: Implementation & Feedback Loops
The final step is about turning decisions into action. Mindhive’s platform allows for the immediate assignment of tasks and responsibilities, ensuring teams can begin executing without lag. Additionally, post-discussion feedback loops enable continuous improvement of both the methodology and the platform. Studies in continuous improvement processes (Deming, Out of the Crisis, 1982) confirm that iterative feedback fosters a learning culture, allowing organizations to refine their collaboration processes over time.
By following these structured steps, The Mindhive Way moves beyond simple brainstorming. It is a holistic, evidence-based framework for turning collective intelligence into tangible, data-driven results in a short window of time.
6. Real-World Applications and Case Scenarios
Innovation Labs: Tech companies can use Mindhive to gather engineering, design, and user research teams in one digital space. Ideas are surfaced quickly, technical feasibility is assessed, and a final prototype direction is chosen—all underpinned by evidence from market research and user feedback.
Healthcare Policy Discussions: Hospitals and research institutions can leverage Mindhive for multi-departmental planning—e.g., optimizing patient flow, improving telemedicine offerings, or aligning on best practices for clinical procedures. The AI ensures all relevant medical studies are cited, helping maintain compliance with regulatory standards.
Government & Public Sector: Mindhive is particularly relevant for public consultations, where a wide range of stakeholders—public servants, community members, NGOs—must reach an agreement on policy issues. AI-driven consensus-building helps reduce bureaucratic gridlocks while ensuring diverse voices are heard.
Corporate Mergers & Acquisitions: When aligning corporate cultures and operational processes post-acquisition, Mindhive can streamline the integration roadmap, highlight critical alignment areas, and track progress to unify teams under a common strategy.
Disaster Response & Crisis Management: Time is of the essence when coordinating relief efforts. Mindhive can integrate real-time data about resource availability, on-ground conditions, and logistical challenges to help diverse agencies converge on prioritized, actionable steps faster.
In each scenario, the versatility of Mindhive’s AI agents, structured discussions, and immediate reporting fosters a collaborative environment where speed and quality of decisions are not mutually exclusive but rather mutually reinforcing.
7. Overcoming Common Objections
“Will AI Replace Human Judgment?”
While Mindhive’s technology automates data gathering, highlighting, and summarization, the final decisions remain squarely in human hands. The platform is a facilitator, not a replacement for critical thinking, creative problem-solving, or ethical deliberations.“Isn’t This Just Another Tool to Learn?”
Mindhive’s user-friendly interface and drag-and-drop features significantly lower the learning curve. Additionally, comprehensive onboarding and dedicated support ensure that even non-technical teams can quickly adopt the platform.“Can We Trust the AI’s Data?”
With source citations built into each AI-generated output, users can verify the credibility and relevance of the information themselves. Moreover, Mindhive adheres to rigorous ISO 27001, GDPR, and SOC2 Type 1 standards, ensuring top-tier data security and ethical handling of information.“What About Organizational Resistance?”
Resistance to change is natural. However, research in change management (Kotter, Harvard Business Review, 1995) shows that seeing immediate benefits—like faster decisions, reduced meeting fatigue, and clearer outcomes—can quickly convert skeptics into advocates.“Will a Rapid Process Sacrifice Thoroughness?”
The structured methodology ensures no critical step is overlooked. Each phase has built-in checks (e.g., data validation, voting, weighting) that mitigate the risk of impulsive or poorly informed decisions.
By directly addressing these concerns, organizations can adopt Mindhive with confidence, secure in the knowledge that speed and due diligence are both upheld through a robust, evidence-based approach.
Conclusion
Modern organizations face an extraordinary challenge: How to innovate rapidly without compromising the quality or sustainability of their decisions. The Mindhive Way embodies a meticulous, research-backed, and AI-enhanced approach that streamlines collaboration, surfaces critical insights, and fosters genuine engagement among participants.
From corporate strategy sessions and public policy consultations to healthcare innovations and disaster response, Mindhive’s structured processes and automated reporting provide a clear path to well-informed, real-time consensus. Drawing on established theories in collective intelligence, cognitive psychology, and organizational behavior, Mindhive ensures that human judgment remains at the center of every decision—while AI agents handle data-intensive tasks that can bog down traditional brainstorming sessions.
As the pace of global business and societal change continues to accelerate, the ability to move from problem to solution in a matter of minutes or hours can be the difference between leading the pack and falling behind. By integrating AI and human insights in an ethical, transparent, and scientifically grounded manner, Mindhive is redefining collaborative decision-making for the 21st century.
Whether you’re a seasoned executive, an innovation lead, or a public policy official, we invite you to explore The Mindhive Way and discover how rapid, evidence-based consensus can unlock the full potential of your collective intelligence.
References & Further Reading
Clark, A. & Chalmers, D. (1998). The Extended Mind. Analysis, 58(1), 7–19.
Chen, L., et al. (2020). Transparent Information Retrieval. ACM Transactions on Information Systems, 38(2), 12–25.
Locke, E. A., & Latham, G. P. (1990). A Theory of Goal Setting & Task Performance. Prentice Hall.
Saaty, T. L. (1990). Decision Making for Leaders. RWS Publications.
Sawyer, R. K. (2007). Group Genius: The Creative Power of Collaboration. Basic Books.
Sweller, J. (1988). Cognitive load during problem-solving: Effects on learning. Cognitive Science, 12(2), 257–285.
Kotter, J. P. (1995). Leading change: Why transformation efforts fail. Harvard Business Review, 73(2), 59–67.
Rock, D. (2008). SCARF: A Brain-Based Model for Collaborating With and Influencing Others. Strategy+Business, 43, 1–9.